3 Magnetic Resonance Imaging
Ethan Draper
Learning Objectives
- Describe a limitation of fMRI.
- Compare and contrast the use of DTI and fMRI.
Magnetic resonance imaging (MRI) is a method of neuroimaging that allows researchers to explore the brain in vivo (in a living organism). Participants lay in a scanner that captures images through two main modalities: structural MRI and functional MRI.
Structural MRIs provide information about anatomy by finding boundaries of structures. Through segmenting different types of tissues (white matter, grey matter, cerebrospinal fluid or CSF), structural MRIs determine the shape and size of different structures in the brain (Soares et al., 2016). This can help neuroscientists locate an individual’s brain lesion and identify other structural abnormalities. One type of structural MRIs are diffusion MRIs (dMRIs). dMRIs provide information about tissue microstructure. While dMRI does not provide direct measures axon size or myelination, some dMRI measures are correlated with these parameters under certain circumstances. dMRI also is useful for tracing white matter fiber bundles, which tells us how different regions are connected to others (Soares et al., 2016). This is referred to as anatomical connectivity. This is pertinent if we are interested in understanding the progressive degeneration of white matter tracts in conditions like multiple sclerosis (MS).
fMRI has two subtypes: task state fMRI and resting state fMRI. Task state involves someone conducting a task (e.g., cognitive task or motor task) in the scanner while images are being acquired. This allows us to connect brain activation patterns with the task. Further clarification about task types will be discussed in section 1.5. Resting state fMRI often involves asking individuals to stare at a cross on a screen and records the brain’s spontaneous activity when not doing a task (Soares et al., 2016). While task fMRI tells us what regions are activated during a task, resting state fMRI tells us what regions are interacting when the brain isn’t doing a specific task.
The Machine
The magnet is always on! To acquire MRIs, participants lay in a large scanner. MRI is non-invasive, however, there are numerous alternative reasons why this setup may not be viable for a given participant. MRI machines are incredibly large, but the space for participants to lay is very tight (as seen in Fig 1). Therefore, it is important to verify if a participant may suffer from claustrophobia prior to scanning. Additionally, given the very strong magnetic field, individuals with some metal or electronic implants (e.g., pacemakers, aneurysm clips, surgical hearing devices, etc.) should not be scanned for safety reasons.
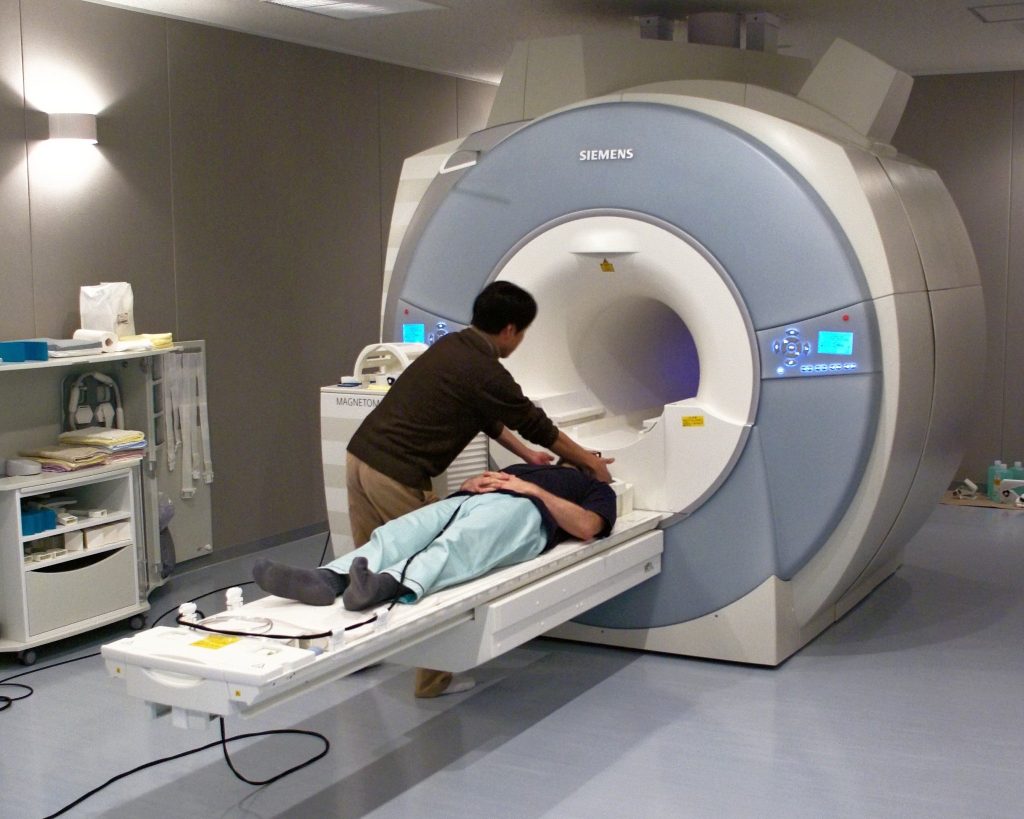
Principles of the MR Signal
Like the rest of the human body, the brain is made up of a large quantity of water. Each water molecule contains two hydrogen molecules which randomly spin. When a participant lays in a scanner, the MRI scanner magnet produces a homogeneous static field (Hidalgo-Tobon, 2010). The randomly spinning hydrogen protons in the brain line up in a predictable manner (van Geuns et al., 1999). Once these are lined up, radio waves are turned on and then systematically removed. When these waves are removed, the magnetization of the protons relaxes. In doing so, they release signals that are recorded by the machine’s radio frequency coils (van Geuns et al., 1999). This signal is used to generate the MR image.
A magnetic gradient is added to the main magnetic field to record each individual brain tissue slice. Repeating this process with different orientations and intensities creates a grid of raw data (called k-space) (van Geuns et al., 1999). The generated k-space is a set of numbers that act as a deconstructed representation of the spatial frequencies of the MR image (Elster, 2021). Using the Fourier transform (a mathematical procedure), k-space data can be transformed into images (Elster, 2021).
fMRI
The Physiological Basis of fMRI
On average, the brain makes up for 5% of a human’s body weight but it uses 20% of the body’s energy. This is because neural activity is incredibly demanding from a metabolic perspective. As such, a well-established relationship exists between energy metabolism and blood supply in the brain that fMRI capitalizes on to acquire its images.
During a task, oxygenated blood rushes to the brain to supply regions with enough nutrients to meet the energy demands of increased brain activity (Nippert et al., 2018). This relationship is called neurovascular coupling (De Silva and Faraci, 2017). Because hemoglobin (the primary carrier of oxygen) is diamagnetic (very slightly repelled by the magnetic field) when oxygenated and paramagnetic (attracted to the magnetic field) when deoxygenated, changes in the blood-oxygen-level-dependent (BOLD) signal are detectable by fMRI (Buxton et al., 2004). The decay rate of the magnetic signal is increased by deoxyhemoglobin in blood vessels (Buxton, 2013). Therefore, the influx of oxygenated blood from activity leads to an increased ratio of oxy- to deoxy-genated hemoglobin, giving it a stronger signal compared to surrounding tissue.
But does the BOLD signal look the same in everyone? Gauthier et al. (2013) asked an important question: can we interpret fMRI results for older and younger individuals equally? The argument is made that metabolic and vascular changes associated with aging will influence the coupling between the neuronal activity and the hemodynamic response that is picked up by the BOLD signal. Evidence suggests that BOLD responses may represent a larger change in neuronal activity in older individuals than the same BOLD response in a younger individual.
Further, it is important to ask if the changes observed in fMRI can be observed in all tissue types? The answer to that question has been an ongoing debate in the field of cognitive neuroscience. fMRI has previously been assumed to be insufficient to detect white matter activation, and only used to explore the neuronal-dense grey matter regions. However, Gawryluk et al. (2014) has made a case for the use of fMRI to study white matter by presenting evidence of hemodynamic changes during various tasks. However, much work remains to be done to understand the relationship between structural white matter changes and functional impairments, as well as improving the assessment and diagnosis of connectivity disorders (Gawryluk et al., 2014).
Acquisition and Experimental Design
When conducting an fMRI study, the most suitable approach depends on the research question and purpose. When the research question is related to a cognitive process, various paradigms can be used to present the associated stimuli. Given their ease, the most studied stimuli are visual stimuli. fMRI scanners are noisy, making them ill-suited for auditory stimuli. Additionally, the goal is to acquire images of the brain that are as clear as possible. As such, restricting movement is very important; this excludes a large majority of motor activities as well.
Stimuli can be presented in what is called a block design. Block designs involve the presentation of stimuli sequentially within a condition (Amaro & Barker, 2006). In the simplest block designs, the stimuli are presented in alternating epochs of other conditions (e.g., face vs scrambled face). Two epochs (one of each condition) is considered a cycle in this block design (Amaro & Barker, 2006).
Alternatively, event related designs may also be used. These involve separating the elements of an experiment into discrete points in time. Different options may be presented, with the participant indicating a response through a scanner safe button (e.g., a stimulus discrimination task). Paring this with the now separated elements of an experiment into distinct time points facilitates the independent analysis of each physiological response to the stimuli (Huettel, 2012).
Oftentimes, both resting state and task fMRI images will be acquired. When doing so it is recommended to acquire the resting state data first. This is to prevent any residual activation in the brain resulting from the task session (Soares et al., 2016).
Analysis and Software Packages
An important concept in fMRI analysis is cognitive subtraction. This process involves comparing the activation in a region of interest during a task of interest to a control task. For example, an individual may be shown images of faces as part of a facial processing experiment. The activation will be interesting, but ultimately meaningless unless it can be shown that the recorded activation is associated with the task and is not merely intrinsic activity. As such, the participant may also be shown non-facial visual stimuli (e.g., a picture of fruit) or of a warped/scrambled version of facial stimuli. Next, the fMRI signal recorded while the participant completed the facial processing task will be subtracted from the control visual task. This resulting activity is the difference observed by the facial processing task and is the activity of interest. Some software that is often used for this analysis is BrainVoyager and FSL.
Applications and Limitations of fMRI
fMRI is very helpful for localizing regions during activity and exploring neuroplasticity (changes to and reorganization of the nervous system; Soares et al., 2016). However, what is its clinical utility? Currently, there is only one clinical use of fMRI: presurgical mapping. When an individual requires neurosurgery, presurgical mapping is conducted to identify functional regions bordering a tumor (Stevens et al., 2016). This is pertinent for optimizing surgical planning and reducing likelihood of a surgeon removing functionally necessary tissue.
It is important to recognize that there are numerous challenges and limitations of fMRI work. fMRI studies frequently have limited sample sizes and a high number of comparisons, meaning they have lower statistical power. One of the main reasons fMRI studies frequently have small sample sizes is that they are very expensive experiments to run. Participants need to be compensated, the MR machine needs to be booked in a slot (which can be hard to do if one scanner is used for both research and clinical purposes), and everyone working on the project needs to be compensated. This includes technicians, the research team, interns, and potentially others depending on the study. As such, there is a growing movement in the field of cognitive neuroscience to share brain imaging data once it has been acquired. This allows more studies to be completed using data, proving to be a more cost-effective and accessible option.
Another limitation of this modality is that fMRI data are highly susceptible to artifacts (discrepancies in the recorded image from the actual participant). These can be caused by metallic objects (e.g., hair ties), hardware malfunctions, or when the scanner doesn’t know where a signal is coming from and misidentifies the signal as coming from two places (a process known as ghosting).
Principles of Diffusion Tensor Imaging (DTI)
What is Diffusion?
Diffusion tensor imaging (DTI) is another noninvasive neuroimaging tool. This variant of diffusion-weighted imaging (DWI) MRI produces images by recording tissue water diffusion rates (Ranzenberger & Snyder, 2022). DWI refers to the contrast of the images, whereas DTI refers to the type of modeling applied to the images that measures the degree of anisotropy and structural orientation.
Water molecules are profuse in the brain. What makes them interesting to examine is that their diffusion is heterogeneous. Depending on the type of tissue and architectural differences, water molecules in the brain diffuse differently. As such, we can infer various properties at the voxel level, including the molecular diffusion rate (Mean Diffusivity; MD) and we can evaluate white matter fiber tracts (Fractional Anisotropy; FA(Kaplan, 2011) though not the direction of the bundles.
Axons measure approximately 1/1000 mm in width. Currently we do not have the technology to image individual axons, but with DTI we can image groups of axons that form white matter bundles.
There are different ways water molecules can move. If the molecules are moving equally in all directions, this is known as isotropic diffusion. Conversely, anisotropic diffusion refers to water molecules moving in a directional way instead of randomly. This tends to occur along white matter fibers (Ranzenberger & Snyder, 2022). The use of individual voxels’ directional information paired with anisotropic diffusion allows the reconstruction of white matter tracts that can then inform neuroscientists about the underlying brain structure (Huisman, 2010).
This information can also be used to create colour FA maps which demonstrate the direction of the fibers. In these graphical depictions of white matter connectivity, brighter colours represent white matter bundles with greater anisotropic diffusion. Most commonly, red fiber tracts are left to right, green are front to back, and blue are up and down.
DTI can also be used to generate tractograms (see Fig 2) and MD maps.
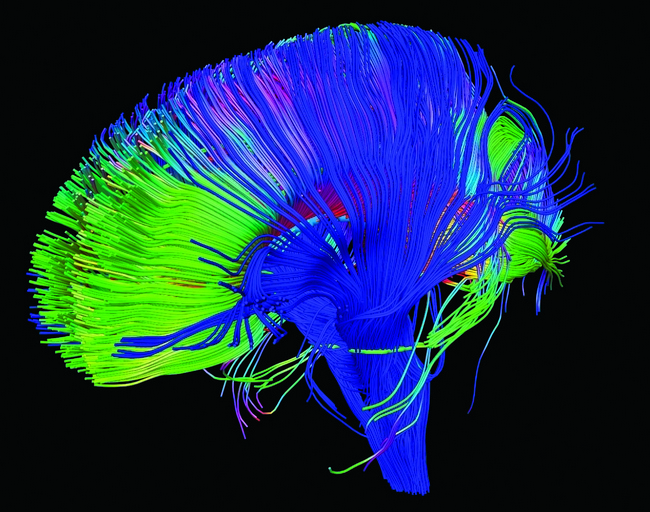
Applications and Limitations of DTI
DTI is often used to explore diseases associated with white matter dysconnectivity. This includes traumatic brain injuries (TBIs), multiple sclerosis (MS), amyotrophic lateral sclerosis (ALS), Parkinson’s disease, epilepsy, and stroke (Tae et al., 2018).
While the possibilities of DTI are exciting, this modality is not without its flaws. It currently has a low signal to noise ratio (SNR) and requires long scan times (Huisman, 2010). Further, it has low spatial resolution and is very susceptible to motion (Soares et al., 2013).
References
Amaro, E., & Barker, G. J. (2006). Study design in fMRI: Basic principles. Brain and Cognition, 60(3), 220–232. https://doi.org/10.1016/j.bandc.2005.11.009
FreeSurfer. (n.d.). FreeSurfer. Retrieved March 8, 2023, from https://surfer.nmr.mgh.harvard.edu
Gauthier, C. J., Madjar, C., Desjardins-Crépeau, L., Bellec, P., Bherer, L., & Hoge, R. D. (2013). Age dependence of hemodynamic response characteristics in human functional magnetic resonance imaging. Neurobiology of Aging, 34(5), 1469–1485. https://doi.org/10.1016/j.neurobiolaging.2012.11.002
Gawryluk, J. R., Mazerolle, E. L., & D’Arcy, R. C. N. (2014). Does functional MRI detect activation in white matter? A review of emerging evidence, issues, and future directions. Frontiers in Neuroscience, 8, 239. https://doi.org/10.3389/fnins.2014.00239
Huettel, S. A. (2012). Event-related fMRI in cognition. Neuroimage, 62(2), 1152–1156. https://doi.org/10.1016/j.neuroimage.2011.08.113
Huisman, T. A. G. M. (2010). Diffusion-weighted and diffusion tensor imaging of the brain, made easy. Cancer Imaging, 10(1A), S163–S171. https://doi.org/10.1102/1470-7330.2010.9023
Kaplan, P. E. (2011). Fractional Anisotropy. In J. S. Kreutzer, J. DeLuca, & B. Caplan (Eds.), Encyclopedia of Clinical Neuropsychology (pp. 1074–1074). Springer. https://doi.org/10.1007/978-0-387-79948-3_32
Le Bihan, D., Mangin, J.-F., Poupon, C., Clark, C. A., Pappata, S., Molko, N., & Chabriat, H. (2001). Diffusion tensor imaging: Concepts and applications. Journal of Magnetic Resonance Imaging, 13(4), 534–546. https://doi.org/10.1002/jmri.1076
Moren, J. (2008). FMRI [Photo]. https://www.flickr.com/photos/jannem/6278833383/
NICHD. (2012). Neural pathways in the brain [Photo]. https://www.flickr.com/photos/nichd/16672073333/
Ranzenberger, L. R., & Snyder, T. (2022). Diffusion Tensor Imaging. In StatPearls. StatPearls Publishing. http://www.ncbi.nlm.nih.gov/books/NBK537361/
Soares, J. M., Magalhães, R., Moreira, P. S., Sousa, A., Ganz, E., Sampaio, A., Alves, V., Marques, P., & Sousa, N. (2016). A hitchhiker’s guide to functional magnetic resonance imaging. Frontiers in Neuroscience, 10. https://doi.org/10.3389/fnins.2016.00515
Soares, J. M., Marques, P., Alves, V., & Sousa, N. (2013). A hitchhiker’s guide to diffusion tensor imaging. Frontiers in Neuroscience, 7. https://doi.org/10.3389/fnins.2013.00031
Stevens, M. T. R., Clarke, D. B., Stroink, G., Beyea, S. D., & D’Arcy, R. C. (2016). Improving fMRI reliability in presurgical mapping for brain tumours. Journal of Neurology, Neurosurgery and Psychiatry, 87(3), 267. https://doi.org/10.1136/jnnp-2015-310307
Tae, W.-S., Ham, B.-J., Pyun, S.-B., Kang, S.-H., & Kim, B.-J. (2018). Current Clinical Applications of Diffusion-Tensor Imaging in Neurological Disorders. Journal of Clinical Neurology (Seoul, Korea), 14(2), 129–140. https://doi.org/10.3988/jcn.2018.14.2.129